Analysis of Determinant Factors of Carbon Efficiency in Indonesia Based on Domestic Waste Management using Causal Machine Learning
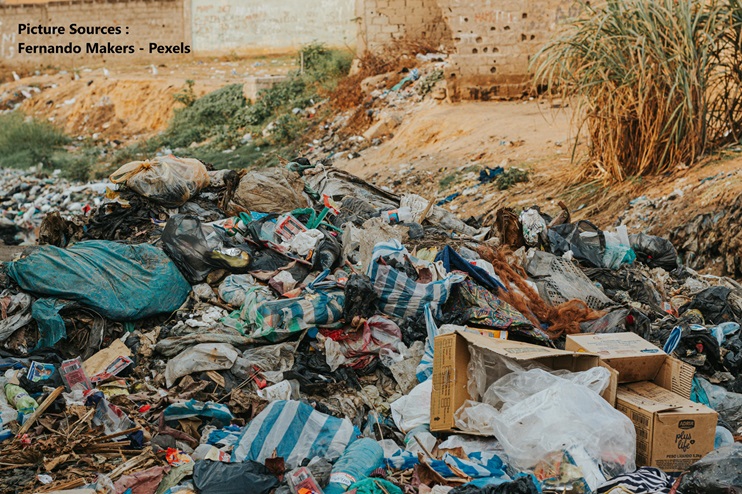
Introduction: Domestic waste management, which is a provincial-level program, is expected to reduce greenhouse gas (GHG) emissions for the sustainability of climate control efforts in Indonesia. Given this context, it is necessary to conduct a carbon efficiency analysis in Indonesia based on domestic waste management efforts. Methods: This study used an observational research design with a cross-sectional time approach. This study predicted the reduction in carbon emissions based on domestic waste management using causal machine learning by analyzing data on GHG emissions and domestic waste management from all provinces in Indonesia. An advantage of causal machine learning is its ability to assess the impact of treatment (domestic waste management) on the results (GHG emissions), as well as mitigating the effects of confounding variables. Results and Discussion: Despite improvements in waste management, several provinces experienced increased waste production, particularly from domestic waste and plastic waste. Analysis using the R programming language revealed that waste management is a significant variable (p = 0.011). However, data limitations posed challenges to comprehensive analysis. Conclusion: Achieving carbon efficiency requires serious waste management efforts. All provinces and cities/regencies must actively participate in program implementation. Routine reporting is essential to monitor the progress toward reducing GHG emissions.
Wei R, Hu Y, Yu K, Zhang L, Liu G, Hu C, et al. Assessing the Determinants Of Scale Effects On Carbon Efficiency In China’s Wastewater Treatment Plants Using Causal Machine Learning. Resour, Conserv Recycl. 2024;203(1):1–10. https://doi.org/10.1016/j.resconrec.2024.107432
Choi E, Eum H, Seo YS, Yi SM, Lee H. Variability of Nitrous Oxide and Carbon Dioxide Emissions Continuously Measured In Solid Waste Incinerators. J Mater Cycles Waste Manag. 2018;20(2):832–843 https://link.springer.com/article/10.1007/s10163-017-0644-y
Moreira SLS, dos Santos RAF, de Castro Paes É, Bahia ML, Cerqueira AES, Parreira DS, et al. Carbon Accumulation in The Soil and Biomass of Macauba Palm Commercial Plantations. Biomass and Bioenergy. 2024;190(1):1-12. https://doi.org/10.1016/j.biombioe.2024.107384
Del Hierro AG, Moreno-Cid JA, Casey E. Continuous Biomanufacturing for Sustainable Bioeconomy Applications. EFB Bioeconomy J. 2024;4(1):1-14. https://doi.org/10.1016/j.bioeco.2024.100071
Stewart-Ibarra AM, Romero M, Hinds AQJ, Lowe R, Mahon R, van Meerbeeck CJ, et al. Co-Developing Climate Services For Public Health: Stakeholder Needs and Perceptions For The Prevention and Control Of Aedestransmitted Diseases in the Caribbean. PLoS Negl Trop Dis. 2019;13(10):1–26. https://doi.org/10.1371/journal.pntd.0007772
Zhuang H, Zhang Z, Han J, Cheng F, Li S, Wu H, et al. Stagnating Rice Yields in China Need To Be Overcome By Cultivars and Management Improvements. Agric Syst. 2024;221(1):1-11. https://doi.org/10.1016/j.agsy.2024.104134
Liu K, Huang T, Xia Z, Xia X, Wu R. The Impact Assessment of Low-Carbon City Pilot Policy on Urban Green Innovation: A Batch-Time Heterogeneity Perspective. Appl Energy. 2025;377(1):1-15. https://doi.org/10.1016/j.apenergy.2024.124489
Liu X, Sim J, Raman V, Viollet Y, AlRamadan AS, Cenker E, et al. Computational Investigation of A Methanol Compression Ignition Engine Assisted By A Glow Plug. Int J Engine Res. 2024. https://doi.org/10.1177/14680874241276061
Shahpouri S, Gordon D, Shahbakhti M, Koch CR. Transient NOx Emission Modeling of A Hydrogen-Diesel Engine Using Hybrid Machine Learning Methods. Int J Engine Res. 2024;25(12):2249-2266. https://doi.org/10.1177/14680874241272898
Al-Qubati A, Zhang L, Forkel M. Urban and Peri-Urban Agriculture Under Climate Change: A Review On Carbon Emissions and Sequestration. Sustain Cities Soc. 2024;115(1)1-13. https://doi.org/10.1016/j.scs.2024.105830
Güllü A, Memiş S, Yaprak H, Gencel O, Ozbakkaloglu T. Performance, Cost, and Ecological Assessment of Fiber-Reinforced High-Performance Mortar Incorporating Pumice Powder and Ground Granulated Blast Furnace Slag As Partial Cement Replacement. J Clean Prod. 2024;476(1):1-18. https://doi.org/10.1016/j.jclepro.2024.143720
Liu J, Zhang Y, Liu Z, Leng J, Zhou H, Gu S, et al. Digital Twins Enable Shipbuilding. Alexandria Eng J. 2024;107(1):915–931. https://doi.org/10.1016/j.aej.2024.09.007
San-Juan-Heras R, Gabriel JL, Delgado MM, Alvarez S, Martinez S. Scientometric Analysis of Cover Crop Management: Trends, Networks, and Future Directions. Eur J Agron. 2024;161(1):1-11. https://doi.org/10.1016/j.eja.2024.127355
Mawcha KT, Malinga L, Muir D, Ge J, Ndolo D. Recent Advances in Biopesticide Research and Development: A Focus on Microbial: A Review. F1000Research. 2024;13(1071):1-41. https://doi.org/10.12688/f1000research.154392.1
Kissi SK, Bryant IM, Boateng SN, Antwi GB. Assessment of Ghana’s Municipal Solid Waste for Valorization–a Review. preprints.org. 2024;1(1):1-16. https://www.preprints.org/manuscript/202409.1493
Lima MMC. The Ignored Impact of the Livestock Sector on Climate Change: An Analysis from the Perspective of International Law. Ger Law J. 2024;1–17. https://doi.org/10.1017/glj.2024.28
Jha AK, Nandulal IS. Vermicomposting: A Sustainable Solution for Agricultural Waste Management, Unlocking The Value from Waste Approaches in Bio-resource Management. New Delhi: Nipa; 2024. p 87-104.
Pan X, GUAN W, Gu J, Luo S, Wang X, ZHAO HUA, et al. Optimization of the Low-Load Performance and Emission Characteristics for a Heavy-Duty Diesel Engine Fueled with Diesel/Methanol by Rsm-Nswoa. SSRN. 2024;1-80. https://dx.doi.org/10.2139/ssrn.4960033
Chen Y, Deng C, Zhu J, Du B, Zhang R, Li Z, et al. Gasification for SNG-power Cogeneration Using Coal as Material Integrated with Calcium Looping for Enhancing CO2 Absorption. ChemRxiv. 2024;1(1):1-36. https://doi.org/10.26434/chemrxiv-2024-grhpq
Zou L, Guo S, Feng Z, Shao H, He X, Wu A, et al. The Effects of Conventional and Microwave Torrefaction on Waste Distiller’s Grains and Its Steam Gasification Characteristics. Fuel. 2024;380(1):1-14. https://doi.org/10.1016/j.fuel.2024.133163
Behroozeh S, Hayati D, Karami E, Nassiri SM, Rezaei-Moghaddam K. Evaluation and Comparison of Energy Use Efficiency Among Cucumber Greenhouses. Front Sustain Food Syst. 2024;8(1):1-14. https://doi.org/10.3389/fsufs.2024.1427530
Tri N, Anh NP, Long DB, Thanh HGT, Nhung BTH, Van NTT, et al. Advanced Zr-CeO2 Supported Ni Catalysts for Production of Synthetic Natural Gas (SNG) from CO2. Fuel. 2025;380(1):1-15. https://doi.org/10.1016/j.fuel.2024.133137
Zahariev A, Barbu A-LMC-M, Dalay BKI, Radjenovic SST, Mihaylova PAM, Deatcu-Gavril C-EPC, et al. Handbook on Circular Economy Challenges for Business and Society. Bulgaria: Tsenov Academic Publishing House; 2024.
Liland JJ. Biobased Building Materials’ Contribution to a Circular Economy. Norwegia: Norwegian University of Life Sciences; 2024. https://hdl.handle.net/11250/3153102
Diaconu C-A, Heidler K, Bamber JL, Zekollari H. Multi-Sensor Deep Learning for Glacier Mapping. arXiv240912034. 2024; https://doi.org/10.48550/arXiv.2409.12034
Oliveira RD, da Silva Oliveira LC, Fontes ATM, Ronceros NG, de Jesus AB, Carneiro PSZ, et al. Os Efeitos Do Aquecimento Global Na Ampliação Das Áreas Desertificadas: Um Estudo Acerca Da Geografia Econômica Dos Municípios Do Sertão Da Bahia E Possíveis Mitigações Da Teoria Da Tríplice Hélice. Contrib A LAS CIENCIAS Soc. 2024;17(9):e10671–e10671. https://dx.doi.org/10.55905/revconv.17n.9-221
Jayakarthik R, Chandrashekhara KT, Sampath O, Kumar D, Biban L, Maroor JP, et al. Climate Impact Prediction: Whale-Optimized Conv-XGBoost with Remote Sensing and Sociological Data. Remote Sens Earth Syst Sci. 2024;7(1):443-456. https://doi.org/10.1007/s41976-024-00136-w
Wang H, Wang X, Yin Y, Deng X, Umair M. Evaluation of Urban Transportation Carbon Footprint−Artificial Intelligence Based Solution. Transp Res Part D Transp Environ. 2024;136(1):1-17. https://doi.org/10.1016/j.trd.2024.104406
Magazzino C, Falcone PM. Assessing The Relationship Among Waste Generation, Wealth, And GHG Emissions In Switzerland: Some Policy Proposals For The Optimization Of The Municipal Solid Waste In A Circular Economy Perspective. J Clean Prod. 2022;351(1):1-14. https://doi.org/10.1016/j.jclepro.2022.131555
Liu J, Zheng L. Structure Characteristics and Development Sustainability of Municipal Solid Waste Treatment in China. Ecol Indic. 2023;152(1):1-7. https://doi.org/10.1016/j.ecolind.2023.110391
Bian R, Zhang T, Zhao F, Chen J, Liang C, Li W, et al. Greenhouse Gas Emissions from Waste Sectors In China During 2006–2019: Implications For Carbon Mitigation. Process Saf Environ Prot. 2022;161(1):488–497. https://doi.org/10.1016/j.psep.2022.03.050
Du M, Peng C, Wang X, Chen H, Wang M, Zhu Q. Quantification of Methane Emissions from Municipal Solid Waste Landfills in China During The Past Decade. Renew Sustain Energy Rev. 2017;78(1):272–279. https://doi.org/10.1016/j.rser.2017.04.082
Lou Z, Cai B-F, Zhu N, Zhao Y, Geng Y, Yu B, et al. Greenhouse Gas Emission Inventories From Waste Sector In China During 1949–2013 and Its Mitigation Potential. J Clean Prod. 2017;157(1):118–124. https://doi.org/10.1016/j.jclepro.2017.04.135
Liu Y, Chen S, Chen AJY, Lou Z. Variations of GHG Emission Patterns From Waste Disposal Processes In Megacity Shanghai from 2005 to 2015. J Clean Prod. 2021;295(1):1-11. https://doi.org/10.1016/j.jclepro.2021.126338
Liu B, Cao X, Pei J, Feng Z, Liang X. Prediction of Carbon Emissions from Municipal Solid Waste Treatment In 31 Provinces and Cities in China Under the Shared Socio-Economic Pathways. Atmos Pollut Res. 2024;15(1):1-12. https://www.sciencedirect.com/science/article/pii/S1309104223003343
Ahsanti A, Husen A. Pengelolaan Sampah Berbasis Masyarakat dalam Mitigasi Perubahan Iklim: Suatu Telaah Sistematik. J Green Growth dan Manaj Lingkung. 2022;11(1):19–26. https://journal.unj.ac.id/unj/index.php/jgg/article/download/19276/12002
Aprilia A, Tezuka T, Spaargaren G. Inorganic and Hazardous Solid Waste Management: Current Status and Challenges for Indonesia. Procedia Environmental Sciences. 2013;17(1):640–647. https://doi.org/10.1016/j.proenv.2013.02.080
Kanugrahan SP, Hakam DF. Long-Term Scenarios of Indonesia Power Sector to Achieve Nationally Determined Contribution (NDC) 2060. Energies. 2023;16(12):1-23. https://doi.org/10.3390/en16124719
Minister of Environment and Forestry of Republic Indonesia. Achievement Report of Waste Management. Jakarta: Ministry of Environment and Forestry of Republic Indonesia; 2024 https://sipsn.menlhk.go.id/sipsn/
Finanda N, Gunarto T. Analisis Pengaruh Pertumbuhan Ekonomi, Pertumbuhan Penduduk, Serta Tingkat Kemiskinan Terhadap Indeks Kualitas Lingkungan Hidup. J Sos dan Sains. 2022;2(1):193–202. https://doi.org/10.59188/jurnalsosains.v2i1.324
Sharma A, Kiciman E. DoWhy: An End-To-End Library for Causal Inference. arXiv Prepr arXiv201104216. 2020;11(1):1-5 https://doi.org/10.48550/arXiv.2011.04216
Syrgkanis V, Lewis G, Oprescu M, Hei M, Battocchi K, Dillon E, et al. Causal Inference and Machine Learning In Practice With Econml and Causalml: Industrial Use Cases At Microsoft, Tripadvisor, Uber. In: Proceedings of the 27th ACM SIGKDD Conference on Knowledge Discovery & Data Mining. 2021;27(1):4072–4073. https://doi.org/10.1145/3447548.3470792
Oberthür S, Groen L. Explaining Goal Achievement in International Negotiations: The EU and The Paris Agreement on Climate Change. J Eur Public Policy. 2018;25(5):708–727. https://doi.org/10.1080/13501763.2017.1291708
Bell A, Jones K. Random Effects Modeling of Time-Series Cross-Sectional And Panel Data. Polit Sci Res Methods. 2015;3(1):133–153. https://doi.org/10.1017/psrm.2014.7
Seetaram N, Petit S. Panel Data Analysis. In: Handbook Of Research Methods In Tourism. England: Edward Elgar Publishing; 2012. https://econpapers.repec.org/bookchap/elgeechap/14747_5f7.htm
Rice WL, Mateer TJ, Reigner N, Newman P, Lawhon B, Taff BD. Changes in Recreational Behaviors of Outdoor Enthusiasts During The COVID-19 Pandemic: Analysis Across Urban and Rural Communities. J Urban Ecol. 2020;6(1):1-7. https://doi.org/10.1093/jue/juaa020
Castañeda-Babarro A, Arbillaga-Etxarri A, Gutiérrez-Santamaría B, Coca A. Physical Activity Change During COVID-19 Confinement. Int J Environ Res Public Health. 2020;17(18):1-10. https://doi.org/10.3390/ijerph17186878
Merta IN, Suderana IW. COVID-19 Pandemic Handling Community Social and Cultural Sector Stimulus Efforts. Int J Soc Sci Humanit. 2020;4(3):1–12. https://dx.doi.org/10.29332/ijssh.v4n3.434
Liou Y-A, Vo T-H, Nguyen K-A, Terry JP. Air Quality Improvement Following COVID-19 Lockdown Measures and Projected Benefits for Environmental Health. Remote Sens. 2023;15(2):1-40. https://doi.org/10.3390/rs15020530
Siddique A, Shahzad A, Lawler J, Mahmoud KA, Lee DS, Ali N, et al. Unprecedented Environmental and Energy Impacts and Challenges Of COVID-19 Pandemic. Environ Res. 2021;193(1):1-12. https://doi.org/10.1016/j.envres.2020.110443
Loh HC, Looi I, Ch’ng ASH, Goh KW, Ming LC, Ang KH. Positive Global Environmental Impacts of the COVID-19 Pandemic Lockdown: A Review. GeoJournal. 2021;87(1):4425–4437. https://doi.org/10.1007/s10708-021-10475-6
Nundy S, Ghosh A, Mesloub A, Albaqawy GA, Alnaim MM. Impact Of COVID-19 Pandemic on Socio-Economic, Energy-Environment and Transport Sector Globally and Sustainable Development Goal (SDG). J Clean Prod. 2021;312(1):1-24. https://doi.org/10.1016/j.jclepro.2021.127705
Moriarty P, Honnery D. New Approaches for Ecological and Social Sustainability In A Post-Pandemic World. World. 2020;1(3):191–204. https://doi.org/10.3390/world1030014
President of Republic Indonesia. President Regulation No. 97 Year 2017 about Regional Policies and Strategies for Management of Household Waste and Similar Types of Household Waste. Jakarta: Ministry of State Secretariat Republic of Indonesia; 2017. https://peraturan.bpk.go.id/Details/73225/perpres-no-97-tahun-2017
SW Indonesia. Indonesia’s Remarkable Expansion: 37 Provinces and Driving Equitable Development. Jakarta: SW Indonesia; 2022. https://sw-indonesia.com/insights/miscellaneous/indonesia-has-37-provinces/
World Bank Group. Global Waste to Grow by 70 Percent by 2050 Unless Urgent Action is Taken: World Bank Report. PRESS RELEASE NO: 2018/037/SURR. Washington DC: World Bank Group; 2018.
Suryani R, Sa’adah N, Lubis I. The Processing of Household Waste by Residents of Griya Serpong Sub-District, South Tangerang, Indonesia, in Compliance with Law Number 18 of 2008 and Law Number 32 of 2018. Sinergi Int J Law. 2023;1(3):205–213. https://doi.org/10.61194/law.v1i3.95
Wiryono S, Gatra S. Sepanjang 2019 Terjadi 733 Kali Gempa Bumi di Banten dan Sekitarnya. Kompas. 2020:1(1). https://megapolitan.kompas.com/read/2020/01/06/18313491/sepanjang-2019-terjadi-733-kali-gempa-bumi-di-banten-dan-sekitarnya
Staley S. The Link Between Plastic Use and Climate Change: Nitty-gritty. California: Stanford Magazine; 2009. https://stanfordmag.org/contents/the-link-between-plastic-use-and-climate-change-nitty-gritty
Stegmann P, Daioglou V, Londo M, van Vuuren DP, Junginger M. Plastic Futures And Their CO2 Emissions. Nature. 2022;612(1):272–276. https://doi.org/10.1038/s41586-022-05422-5
Dietplastik Indonesia. Pelarangan Kantong Plastik di Provinsi DKI Jakarta. Jakarta: Dietplastik Indonesia; 2020. https://plasticdiet.id/pelarangan-kantong-plastik-di-provinsi-dki-jakarta/
Helakombo H, Widya Yanti DI, Masengi MC, Tabalessy RR, Manurung M. Identifikasi Jenis Sampah Laut (Marine Debris) di Pantai Tanjung Saukabu, Kecamatan Waigeo Barat Kepulauan Kab. Raja Ampat Provinsi Papua Barat Daya. Innov J Soc Sci Res. 2024;4(4):7270-7280. https://j-innovative.org/index.php/Innovative/article/view/12939
Seadon J, Ala’Aladwan AUT, Golding K. Mandatory Site Waste Management Plans. San Fransisco: Auckland University of Technology; 2022.
Windfeld ES, Brooks MS-L. Medical Waste Management: A Review. J Environ Manage. 2015;163(1):98–108. https://doi.org/10.1016/j.jenvman.2015.08.013
Zarrinpoor N. A Sustainable Medical Waste Management System Design In The Face Of Uncertainty And Risk During COVID-19. Fuzzy Optim Decis Mak. 2023;22(3):519–554. https://doi.org/10.1007/s10700-022-09401-3

This work is licensed under a Creative Commons Attribution-NonCommercial-ShareAlike 4.0 International License.
1. Copyright of all journal manuscripts is held by the Jurnal Kesehatan Lingkungan.2. Formal legal provisions to access digital articles of electronic journal are subject to the provision of the Creative Commons Attribution-ShareAlike license (CC BY-NC-SA), which means that Jurnal Kesehatan Lingkungan is rightful to keep, transfer media/format, manage in the form of databases, maintain, and publish articles.
3. Published manuscripts both printed and electronic are open access for educational, research, and library purposes. Additionally, the editorial board is not responsible for any violations of copyright law.
JKESLING by UNAIR is licensed under a Creative Commons Attribution-ShareAlike 4.0 International License.