THE ROLE OF ARTIFICIAL INTELLIGENCE (AI) ON MRI BRAIN EXAMINATION WITH CLINICAL ISCHEMIC STROKE
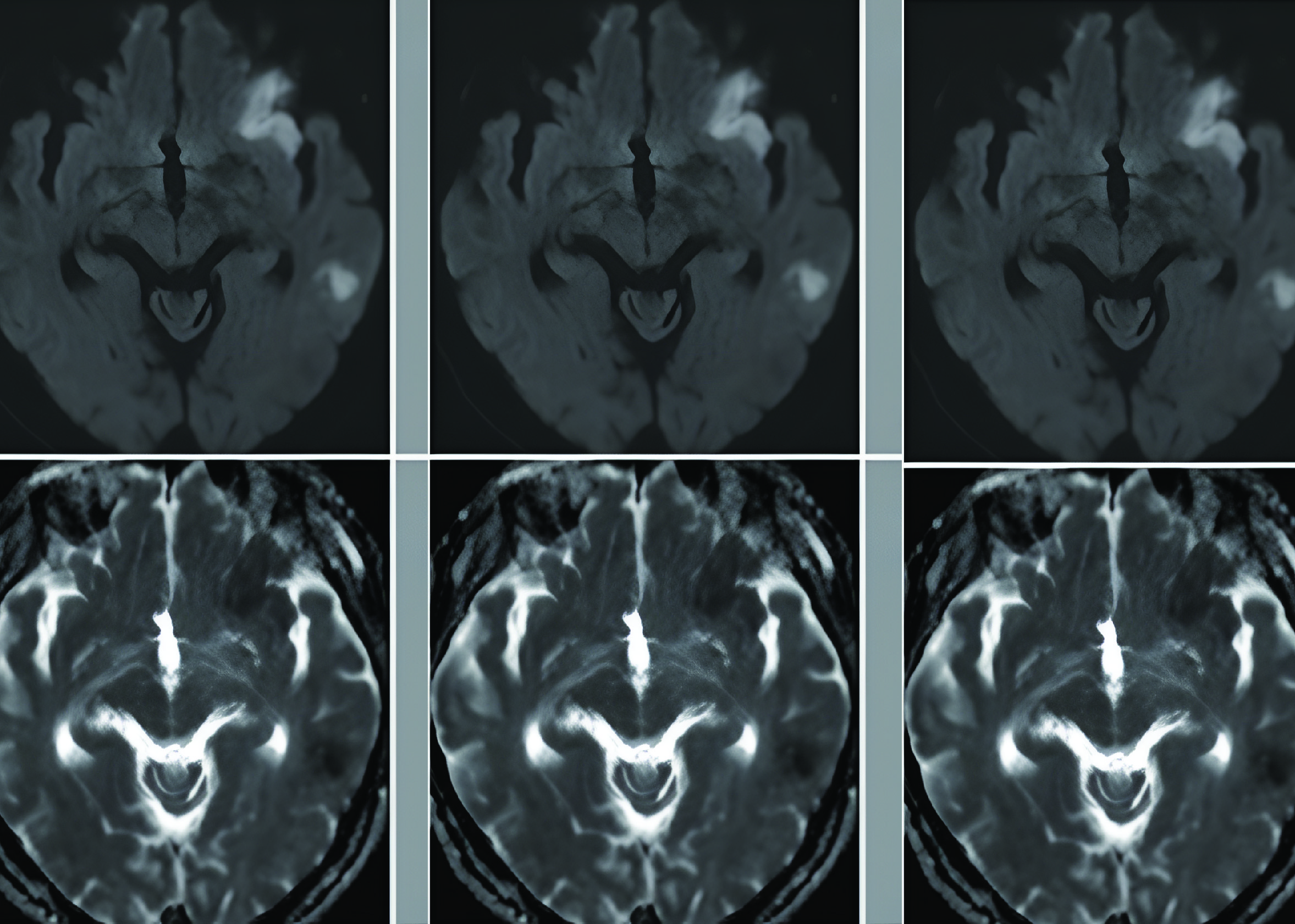
Downloads
Background: Application of Artificial Intelligence (AI) in radiology is named automatic image interpretation of neuroimaging stroke. It takes a short time to minimize the patient's brain damage. Purpose: Determine the role of AI in ischemic brain stroke MRI examination and find out the advantages and disadvantages of applying AI to ischemic brain stroke MRI examination. Review: It was a descriptive and qualitative study with a literature review approach. The selection of articles used the ScienceDirect, Scopus, ProQuest, PubMed, and Publish or Perish databases. The inclusion criteria included full articles, with the topic of AI on ischemic brain stroke MRI examinations published in the 2017 – 2022 range, articles published by English-language international journals with a classification of Q1 – Q3, and having DOI. Seven relevant pieces of article were obtained, then descriptive analysis was carried out by comparing and presenting the articles descriptively in tabular form. Result: The role of AI in MRI brain examination with clinical ischemic stroke, namely its role in automatic lesion segmentation, Time Since Stroke (TSS) classification, and infarct volume prediction. The advantages of AI included short image processing times and accurate results. The disadvantages of AI tended to decrease performance in small lesions, a large number of patients, limited data, and false positive results. The value of the Dice Score Coefficient (DSC) (0.53 – 0.86) was already high even though it had not reached 1 because it depended on the strength of the data used. Conclusion: The role of AI in MRI imaging of ischemic brain stroke helps in the diagnosis and prognosis of ischemic stroke patients. AI in stroke neuroimaging has advantages in time effectiveness and disadvantages in data limitations.
Arif, M., Okraini, N., Putra, A.Y.M., 2019. Hubungan Ketepatan ‘GOLDEN PERIOD' dengan Derajat Kerusakan Neurologi pada Pasien Stroke Iskemik diruang Instalasi Gawat Darurat Rumah Sakit Stroke Nasional Bukit Tinggi Tahun 2018. Prosding Semin. Kesehat. Perintis Vol. 2(1), Pp. 94.
Benzakoun, J., Charron, S., Turc, G., Hassen, W. Ben, Legrand, L., Boulouis, G., Naggara, O., Baron, J.-C., Thirion, B., Oppenheim, C., 2021. Tissue Outcome Prediction in Hyperacute Ischemic Stroke: Comparison of Machine Learning Models. J. Cereb. Blood Flow Metab. Vol.41(11), Pp. 3085-3096.
Budianto, P., Mirawati, D.K., Prabaningtyas, H., Putra, S.E., 2021. Stroke Iskemik Akut: Dasar dan Klinis.UNS Press, Universitas Sebelas Maret.
Chen, L., Chen, L., Rueckert, D., 2017. Fully Automatic Acute Ischemic Lesion Segmentation in DWI using Convolutional Neural Networks. NeuroImage Clin. Vol. 15, Pp. 633-643.
Ding, L., Liu, C., Li, Z., Wang, Y., 2020. Incorporating Artificial Intelligence into Stroke Care and Research. Stroke Vol. 51(2), Pp. e351–e354.
Han, L., Liu, L., Hao, Y., Zhang, L., 2021. Diagnosis and Treatment Effect of Convolutional Neural Network-Based Magnetic Resonance Image Features on Severe Stroke and Mental State. Contrast Media Mol. Imaging Pp. 1-9.
Hardy, M., Harvey, H., 2020. Artificial Intelligence in Diagnostic Imaging: Impact on the Radiography Profession. Bristish J. Radiol. Vol. 93(11), Pp. 20190840.
Ho, K.C., Speier, W., Zhang, H., Fabien Scalzo, S.E.-S., Arnold, C.W., 2019. A Machine Learning Approach for Classifying Ischemic Stroke Onset Time from Imaging. IEEE Trans. Med. Imaging Vol. 38(7), Pp. 1666-1676.
Kim, P., 2017. MATLAB Deep Learning Machine Learning, Neural Networks and Artificial Intelligence. Apress, Seoul, Soul-t'ukpyolsi, Korea (Republic of).
Lee, H., Lee, E.-J., Ham, S., Lee, H.-B., Lee, J.S., Kwon, S.U., Kim, J.S., Kim, N., Kang, D.-W., 2020. MachineLearning Approach to Identify Stroke within 4.5Hours. Stroke Vol. 51(3), Pp. 860-866.
Lee, S.-A., Jang, J.-W., Park, S.-W., Kim, P.-J., Yeo, N.-Y., Kim, C., Kim, Y., Choi, H.-S., Kim, S., 2022. Indirect Volume Estimation for Acute Ischemic Stroke from Diffusion Weighted Image using Slice Image Segmentation. J. Pers. Med. Vol. 12(4), Pp. 521.
Leonard, Greg, Laurent Elder, Maroussia Levesque, Marshall Smith, Pascal Kropf, Sunisha Neupane, Aaron Martin, Raul Zambrano, and Cheryl Chan. 2018. Toward a Research Agenda Artificial Intelligence and Human Development.
Long, B.W., Rollins, J.H., Smith, B.J., 2022. Merrill's Atlas of Radiographic Positioning and Procedures, 13 th. ed. Elseiver.
Lungren, M.P.M.R.B.E., Ranschaert, E.R., Morozov, S., Algra., P.R., 2019. Artificial Intelligence in Medical Imaging. Cham Springer Nat. Switz. AG.
Mouridsen, K., Thurner, P., Zaharchuk, G., 2020. Artificial Intelligence Applications in Stroke. Stroke Vol. 51(8), Pp. 2573–2579.
Mueller, J.P., Massaron, L., 2023. Artificial Intelligence for Dummies. New Jersey: John Wiley & Sons.
Nielsen, A., Hansen, M.B., Tietze, A., Mouridsen, K., 2018. Prediction of Tissue Outcome and Assessment Of Treatment Effect in Acute Ischemic Stroke using Deep Learning. Stroke Vol. 49(6), PP. 1394-1401.
Runge, V.M., Heverhagen, J.T., 2022. Physics of Clinical MR Taught Through Images, 5 th. ed. Springer.Setiawan, P.A., 2021. Diagnosis dan Tatalaksana Stroke Hemoragik. J. Med. Hutama Vol. 3(1), Pp. 1660-1665.
Shafaat, O., Bernstock, J.D., Shafaat, A., Yedavalli, V.S., Elsayed, G., Gupta, S., Sotoudeh, E., Sair, H.I., Yousem, D.M., Sotoudeh, H., 2021. Leveraging Artificial Intelligence in Ischemic Stroke Imaging. J.Neuroradiol. Vol. 49(4), Pp. 343-351.
Sherwood, L., 2015. Human Physiology: from Cells to System, 9 th. ed. Cengage Learning, Canada.
Sultan, S., Saidi, S., 2021. Limitations of Artificial Intelligence. RIT Dubai.
Tomita, N., Jiang, S., Maeder, M.E., Hassanpour, S., 2020. Automatic Post-Stroke Lesion Segmentation on MR Images using 3D Residual Convolutional Neural Network. Neuroimage Clin. Vol. 27, Pp. 102276.
Wang, K., Shou, Q., Ma, S.J., Liebeskind, D., Qiao, X.J., Saver, J., Salamon, N., Kim, H., Yu, Y., Xie,Y., Zaharchuk, G., Scalzo, F., Wang, D.J.J., 2020.Deep Learning Detection of Penumbral Tissueon Arterial Spin Labelling in Stroke. Stroke Vol. 51(2), Pp. 489-497.
Wei, L., Cao, Y., Zhang, K., Xu, Y., Zhou, X., Meng, J., Shen, A., Ni, J., Yao, J., Shi, L., Zhang, Q., Wang, P., 2021. Prediction of Progression to Severe Stroke in Initially Diagnosed Anterior Circulation Ischemic Cerebral Infarction. Front. Neurol. Vol. 12, Pp. 652757.
Wei, Y.-C., Huang, W.-Y., Jian, C.-Y., Hsu, C.-C.H., Hsu, C.-C., Lin, C.-P., Cheng, C.-T., Chen, Y.-L., Wei, H.-Y., Chen, K.-F., 2022. Semantic Segmentation Guided Detector for Segmentation, Classification, and Lesion Mapping of Acute Ischemic Stroke in MRI Images. NeuroImage Clin. Vol. 35, Pp. 103044.
WHO, 2022. Stroke, Cerebrovascular Accident. WHO East. Mediterr. URL https://www.emro.who.int/health-topics/stroke-cerebrovascular-accident/index.html
Woo, I., Lee, A., Jung, S.C., Lee, H., Kim, N., Cho, S.J., Kim, D., Lee, J., Sunwoo, L., Kang, D.W., 2019. Fully Automatic Segmentation of Acute Ischemic Lesions on Diffusion-Weighted Imaging using Convolutional Neural Networks: Comparison with Conventional Algorithms. Korean J. Radiol. Vol. 20(8), Pp. 1275-1284.
You, S.-H., Kim, B., Kim, B.K., Park, S.E., 2021. Fast MRI in Acute Ischemic Stroke: Applications of MRI Acceleration Techniques for MR-Based Comprehensive Stroke Imaging. Investig. Magn. Reson. Imaging Vol. 25(2), Pp. 81-92.
Yu, Y., Xie, Y., Thamm, T., Gong, E., Ouyang, J., Huang, C., Christensen, S., Marks, M.P., Lansberg, M.G., Albers, G.W., Zaharchuk, G., 2020. Use of Deep Learning to Predict Final Ischemic Stroke Lesions from Initial Magnetic Resonance Imaging. JAMA Netw. Open Vol. 3(3), Pp. e200772.
Zhu, H., Jiang, L., Zhang, H., Luo, L., Chen, Yang, Chen, Yuchen, 2021. An Automatic Machine Learning Approach for Ischemic Stroke Onset Time Identification Based on DWI and FLAIR Imaging. NeuroImage Clin. Vol. 31, Pp. 102744.
Copyright (c) 2024 Journal of Vocational Health Studies

This work is licensed under a Creative Commons Attribution-NonCommercial-ShareAlike 4.0 International License.
- The authors agree to transfer the transfer copyright of the article to the Journal of Vocational Health Studies (JVHS) effective if and when the paper is accepted for publication.
- Legal formal aspect of journal publication accessibility refers to Creative Commons Attribution-NonCommercial-ShareAlike (CC BY-NC-SA), implies that publication can be used for non-commercial purposes in its original form.
- Every publications (printed/electronic) are open access for educational purposes, research, and library. Other that the aims mentioned above, editorial board is not responsible for copyright violation.
Journal of Vocational Health Studies is licensed under a Creative Commons Attribution-NonCommercial-ShareAlike 4.0 International License